How evoML Leverages LLMs to Create Synthetic Data for Fast Prototyping and Better Performance
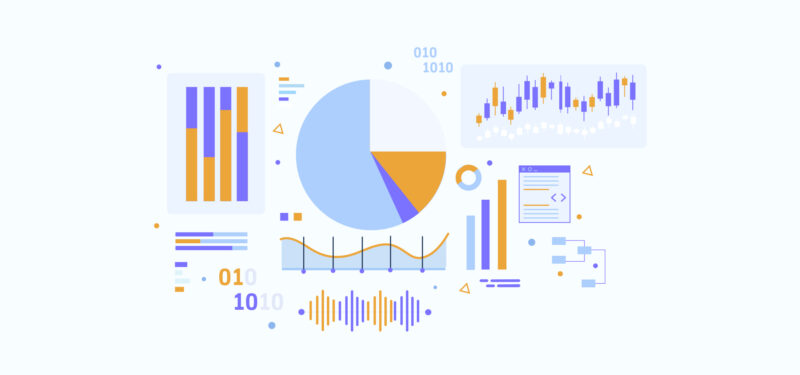
The continuous evolution in the fields of artificial intelligence and machine learning has introduced us to an interesting array of innovations and tools. Among them, Large Language Models (LLMs) are reshaping the boundaries of natural language understanding and generation. Besides their use in chatbots, translators, and other text-based applications, LLMs are also emerging as an effective tool for creating synthetic data. This article looks at how LLMs can be utilised for synthetic data generation and how you can use evoML’s synthetic data generator for fast prototyping.
What is Synthetic Data
Before we delve into how we use LLMs to create synthetic data, it is crucial to understand what synthetic data is. In simple terms, synthetic data is data artificially generated rather than collected from real-world scenarios. Synthetic data is carefully crafted to mimic the properties and statistical behaviour of actual data, ensuring its usefulness in a wide variety of data-hungry tasks, such as model training and performance testing.
The Value of Synthetic Data
Synthetic data has the potential to solve various challenges encountered in the data-driven world. For example, by creating synthetic data that closely resembles the original distribution but does not contain sensitive information, one can perform robust data analysis without violating privacy norms. Moreover, synthetic data can also help overcome data scarcity in specific domains, aiding in better AI model training by providing diversified and balanced datasets. Another benefit of synthetic data is time-saving. Data scientists typically spend over 60% of their time collecting, organising, and cleaning data, rather than on analysis. Synthetic data generation can significantly cut down these efforts, enabling data scientists to spend their time on more critical tasks.
How does evoML Leverage LLMs to Create Synthetic Data?
LLMs are capable of generating human-like text based on the input and training they have received. These models learn from vast quantities of data, encompassing diverse topics and styles, enabling them to generate a wide range of coherent and contextually accurate text.
When applied to synthetic data creation, LLMs can generate data that matches the complexity and variety of real-world data. With an appropriate prompt or seed, an LLM can produce content that mirrors specific characteristics of the target data.
For example, if an LLM is trained on healthcare data and understands the structure of medical records, it could be prompted to generate synthetic medical records. The generated data would have no link to real individuals, thereby maintaining patient privacy, but would be representative of real-world data in its structure and variety, making it invaluable for developing and testing healthcare-related AI applications.
How You Can Use evoML’s Synthetic Data Generation Feature
1. Generating synthetic data for new features based on existing data
evoML leverages LLMs to generate synthetic data for new features based on existing dataset features. This is similar to a predictive model but instead of predicting outcomes, it crafts entirely new datasets.
By analysing patterns and relationships between existing features, evoML's algorithms, backed by LLMs, smartly fabricate new features that are statistically coherent and contextually relevant. Imagine a bank with a vast repository of transactional and customer data. Utilising evoML, the bank can now generate synthetic data to create scenarios for new financial products or services that it plans to introduce. For example, if the bank intends to launch a new type of savings account tailored for young professionals, evoML can simulate data reflecting their unique saving patterns and financial behaviours, based on the existing data of similar customer segments. This synthetic data provides a robust, realistic foundation for testing and refining the new product, ensuring its relevance and appeal to the target demographic. By leveraging this feature, the bank not only enhances its product development process but also significantly reduces the risks and uncertainties associated with introducing new financial products to the market. 2. Identifying relevant data internally for solving particular use cases
Another way we are leveraging LLMs is to generate synthetic datasets custom-made for specific use cases. By synthesizing vast datasets that replicate real-world data contexts and intricacies, evoML offers a sandbox environment for businesses to experiment with and pinpoint the most relevant real datasets they would need for their specific challenges.
Imagine a company wanting to venture into a new market segment, but uncertain about which data would be most suitable to model consumer behaviour within that niche. Using evoML and its underlying LLMs, the company can simulate a synthetic dataset mimicking that market, enabling them to test various analytical models. Through such experimentation, the company can pinpoint which actual data points or features are pivotal to solving their particular use case. In a way, evoML acts as a compass, guiding businesses through the vast sea of data, and helping them find the most pertinent real-world information to anchor their strategies.
Final Thoughts
As we continue to witness the rapid advancements in AI and machine learning, it becomes clear that tools like evoML, powered by LLMs, are not just innovative solutions but essential tools in the quest to harness the full potential of data in our increasingly digital world. The future of data-driven decision-making and AI development seems promising, with LLMs at the forefront, paving the way for more intelligent, efficient, and ethical use of data.